Main Responsibilities and Required Skills for a Data Quality Analyst
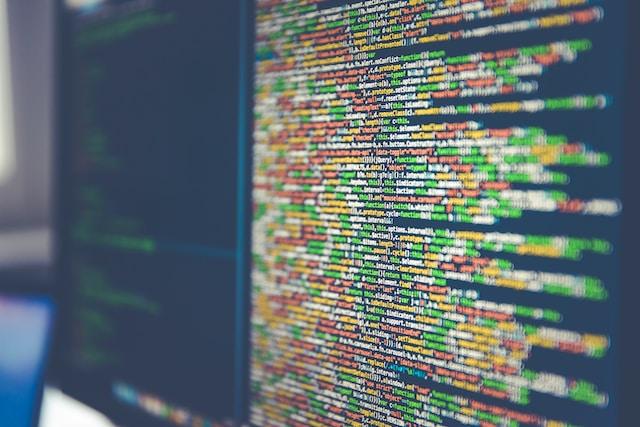
A Data Quality Analyst is a professional who plays a crucial role in ensuring the accuracy, completeness, and reliability of data within an organization. They are responsible for implementing and maintaining data quality standards, identifying data inconsistencies and errors, and developing strategies to improve data quality. In this blog post, we will describe the primary responsibilities and the most in-demand hard and soft skills for Data Quality Analysts.
Get market insights and compare skills for other jobs here.
Main Responsibilities of a Data Quality Analyst
The following list describes the typical responsibilities of a Data Quality Analyst:
Acquire
Acquire a high level of subject matter expertise in order to improve our data.
Acquire data from primary or secondary data sources and organize & maintain databases / data systems.
Adhere to
Adhere to best practices in data analysis and collection.
Analyze
Analyze and identify data redundancies and take steps to correct them.
Analyze data sets to identify data quality issues and anomalies.
Analyze, query and manipulate data according to defined business rules and procedures.
Assess
Assess and report on data quality issues, and to continually enhance the ICES data infrastructure.
Assist in
Assist in development and expansion of various data quality programs and initiatives.
Assist management in defining new process improvement opportunities.
Assist the team in achieving its goals and complete your individual tasks in a timely manner.
Cleanse
Cleanse and correct data errors and inconsistencies.
Collaborate with
Collaborate with cross-functional teams to understand data requirements and resolve quality issues.
Collaborate with data architects to design and implement data quality frameworks and controls.
Collaborate with database developers to implement effective automation processes.
Come up with
Come up with the engineering instructions on how to automate certain manual processes.
Complete
Complete a check and balances Quality review of data abstraction for the pharmacy program areas.
Conduct
Conduct Data Profiling and assessment of Critical Data Elements.
Conduct ongoing monitoring and administration of Master Data Management task backlog.
Conduct regular audits to ensure compliance with data quality standards.
Conduct risk assessments to identify potential data quality vulnerabilities.
Conduct root cause analysis to identify underlying issues causing quality problems.
Consult with
Consult with client data steward when account information is in question.
Contribute to
Contribute to data governance initiatives by defining quality requirements and policies.
Contribute to ongoing process improvement.
Coordinate
Coordinate the planning, documenting, and execution activities for the projects.
Coordinate with different stakeholders to monitor, investigate and troubleshoot data quality issues.
Create
Create and maintain data dictionaries and metadata for efficient data management.
Create and maintain documentation of data, macros, and processes.
Create and maintain reference data in accordance to defined processes and procedures.
Create and maintain SAS macros and other tools to facilitate and standardize data use.
Create Business Requirement specifications to document all data model changes.
Create data quality control assessment and implement any additional controls as required.
Create data quality metrics and scorecards for reporting on data quality.
Create data quality rules assessment and implement any additional rules as required.
Create estimates for DQA work and communicates these to project manager.
Create research-ready, user-friendly, standardized SAS datasets for the ICES Data Repository.
Create Test Check lists, Test Strategy documents.
Define
Define user experience standards and exception reporting capability.
Deliver
Deliver data quality improvement initiatives as defined, agreed and prioritised.
Design
Design and publish data quality dashboards using data visualization tools such as Power BI.
Determine
Determine push / pull model for DQ dashboards (executive dashboards, data remediation dashboards).
Determine root cause for data quality errors and make recommendations for long-term solutions.
Develop
Develop and implement strategies to improve data quality.
Develop and implement test plans and scripts for various data quality processes.
Develop approaches to presenting data to clients that quantifies the power and value of iovation.
Develop archiving and purging strategies and processes.
Develop documentation to support the project planning and implementation process.
Develop tools to track and trend pharmacy errors.
Document
Document all logic and data quality issues.
Drive
Drive efficiency and automation throughout our operations process.
Engage
Engage with colleagues and clients to promote and encourage a collaborative relationship.
Ensure
Ensure adherence to documented data quality standards and measures.
Ensure compliance with data privacy regulations and protect sensitive data.
Ensure data is relevant to executive leadership reports as well as localized program reporting.
Ensure data quality during the integration of data from multiple sources.
Ensure data quality during the migration of data across systems.
Ensure lead data adheres to data quality processes and standards.
Ensure sensitive data is accessed and shared in accordance to defined policies and processes.
Ensure the quality and accuracy of data.
Escalate
Escalate the resolution of all internal data exceptions in timely and accurate manner.
Establish
Establish data quality standards and guidelines for data management processes.
Evolve
Evolve data quality monitoring and trends.
Examine
Examine complex data to optimize efficiency and quality of data collected.
Facilitate
Facilitate elaboration sessions to elicit requirements.
Filter
Filter data by reviewing computer reports, printouts, and key performance indicators.
Follow
Follow defined and agreed Data Quality Issue identification and resolution processes and procedures.
Follow standard practices and procedures when analyzing situations or data.
Generate
Generate regular reports on data quality metrics and performance indicators.
Identify
Identify and document data quality issues for tracking and resolution.
Identify and maintain data quality corrective action plans.
Identify areas of opportunity for reporting and process improvements to drive reporting accuracy.
Identify opportunities for automating data quality processes.
Identify opportunities for improvement in processes.
Identify queries and extract key data.
Implement
Implement quality assurance processes to validate data accuracy and completeness.
Interpret
Interpret and analyze data on a regular basis and provide ongoing reports.
Investigate
Investigate impact of data enrichment and on boarding new data sources.
Investigate root causes of data quality issues and recommend corrective actions.
Lead
Lead development and implementation of automated data cleansing.
Maintain
Maintain a current understanding of Data Governance policies and standards.
Maintain and ensure availability and quality of on-going data feeds.
Maintain and support Data Quality Rules and Preventative / Detective Controls.
Maintain detailed documentation of data quality procedures, protocols, and findings.
Maintain expert level of business functional knowledge for an assigned data domain.
Maintain technical metadata, data lineage, and data governance workflow.
Make
Make recommendations to address quality issues and ensure they are resolved quickly.
Manage
Manage business glossary on data definitions.
Manage Data Access security as needed.
Manage demand while adhering to service level agreements.
Manage quality and maintenance of master data as it is created.
Monitor
Monitor and report on data quality issues.
Monitor data quality for Genesis (Salesforce CRM) and Reporting Platforms.
Monitor data quality metrics and address issues promptly.
Monitor performance, quality control plans, and identify trends or patterns via data analytics.
Monitor QA's to identify any issues or ways to improve systems and database designs.
Optimize
Optimize data quality processes to enhance performance and efficiency.
Own
Own and review processes surrounding various daily / weekly / monthly data reports.
Participate in
Participate actively in the ICES Data Issues Group.
Participate in and possibly lead discussions with cross-functional teams.
Participate in development of best practices and tools based on business needs.
Participate in regular supervision, training, and staff meetings.
Perform
Perform and helps to coordinate chart review activities.
Perform data analysis and transformation using SQL, Excel and tools like Alteryx.
Perform data profiling to assess quality and identify patterns and trends.
Perform other related duties as assigned.
Prepare
Prepare and present analysis results to our various business partner stakeholders.
Present
Present data quality insights and findings in a visually appealing and easy-to-understand manner.
Profile
Profile business, financial and reporting data through use of cutting edge data / analytic tools.
Promote
Promote a high level of data quality awareness across our organization.
Provide
Provide data quality training and presentations to external clients and internal teams.
Provide expert data analysis to the Long Term Care and Retirement Divisions.
Provide guidance and answer questions on data trending.
Provide guidance and support to junior members of the team.
Provide insight and suggestions to improve Data Quality Control Process.
Provide requested data to Law Enforcement while working within company standards and guidelines.
Provide training and guidance on data quality best practices.
Recommend
Recommend creative approaches to structuring and manipulating new data within our platform.
Report
Report progress on problem resolution to management.
Research
Research, correct and enrich lead information where necessary.
Resolve
Resolve all internal data exceptions in a timely and accurate manner.
Review
Review and respond to QC queries to ensure data is accurate.
Review event lists and help identify if relationship already exists with lead.
Review large amount of information in a short time and draw conclusions.
Review lead information for accuracy and completeness.
Review specs and test plans to ensure accuracy and conformance to established standards.
Review, validate, and record metadata and data quality information.
Run
Run data queries to identify coding issues and data exceptions - cleaning data when necessary.
Run reports and collaborate with management on performance against contractual obligations.
Serve
Serve as a data steward by ensuring the integrity and reliability of data.
Set-up
Set-up data quality test and assessment rules.
Share
Share ideas to improve the automation of the insurance fraud investigation process.
Solve
Solve data governance tool related utility problems.
Support
Support archiving and purging activities of data.
Support Data Steward to capture data quality rules and control requirements.
Support metadata repository maintenance, access requests, and proper metadata association.
Support Privacy initiatives through analysis of sensitive data.
Test
Test and review data capture tool with other QA Specialist to ensure data integrity is maintained.
Track
Track and coordinate change order completion, approval, and implementation.
Track lead data metrics including number of new leads.
Track, Monitor, and report on data quality assurance / production performance metrics.
Train
Train colleagues in database software as needed.
Understand
Understand, extract and transform data from CRM applications and its databases.
Understand the impact of our business.
Understand the impacts of timeline delays.
Use
Use of some independent judgment and analysis required.
Utilize
Utilize data cleansing tools and software to improve quality.
Utilize statistical techniques and data analysis tools to identify patterns and trends affecting quality.
Validate
Validate data accuracy through quality checks and validation procedures.
Work with
Work closely with Data Governance Office in establishing enterprise-wide data standards.
Work individually or with teams to develop utilities to prevent, identify and rectify data issues.
Work using Agile methodology to improve the value of data.
Work with management to improve the lead qualifying processes and updates SOP as needed.
Work with management to prioritize business and information needs.
Most In-demand Hard Skills
The following list describes the most required technical skills of a Data Quality Analyst:
SQL (Structured Query Language)
Data Modeling
Data Profiling Tools (e.g., Informatica Data Quality, Talend)
Data Integration Tools (e.g., IBM DataStage, Microsoft SSIS)
Data Analysis and Visualization Tools (e.g., Tableau, Power BI)
Data Cleansing and Data Quality Tools (e.g., Trifacta, Alteryx)
Statistical Analysis and Modeling
ETL (Extract, Transform, Load) Processes
Relational and NoSQL Databases
Data Governance Frameworks
Data Mining Techniques
Scripting Languages (e.g., Python,R, Perl)
Data Warehousing Concepts
Data Migration Techniques
Data Security and Privacy
Data Validation and Testing
Data Quality Assessment Methods
Business Intelligence Tools
Data Governance and Data Management Practices
Proficiency in Microsoft Excel and other spreadsheet software.
Most In-demand Soft Skills
The following list describes the most required soft skills of a Data Quality Analyst:
Attention to Detail: Meticulous in identifying data anomalies and ensuring accuracy.
Analytical Thinking: Ability to analyze complex data sets and identify patterns.
Problem Solving: Skill in identifying and resolving data quality issues.
Communication: Effective communication to collaborate with cross-functional teams and stakeholders.
Collaboration: Ability to work collaboratively in a team environment.
Time Management: Efficiently manage multiple data quality projects and priorities.
Adaptability: Willingness to adapt to evolving data quality requirements and technologies.
Critical Thinking: Apply logical reasoning and critical thinking to data quality challenges.
Continuous Learning: Desire to stay updated with emerging data quality tools and techniques.
Quality Assurance Mindset: Strong commitment to delivering high-quality data and ensuring data integrity.
Conclusion
Being a Data Quality Analyst requires a combination of technical expertise, attention to detail, analytical skills, and effective communication. This role plays a crucial part in maintaining the accuracy and reliability of data, which is essential for making informed business decisions. By fulfilling their responsibilities and possessing the necessary hard and soft skills, Data Quality Analysts contribute significantly to data-driven decision-making and overall organizational success.